Team Led by LSU's Supratik Mukhopadhyay Advances in $11M XPrize Wildfire Competition
April 17, 2024
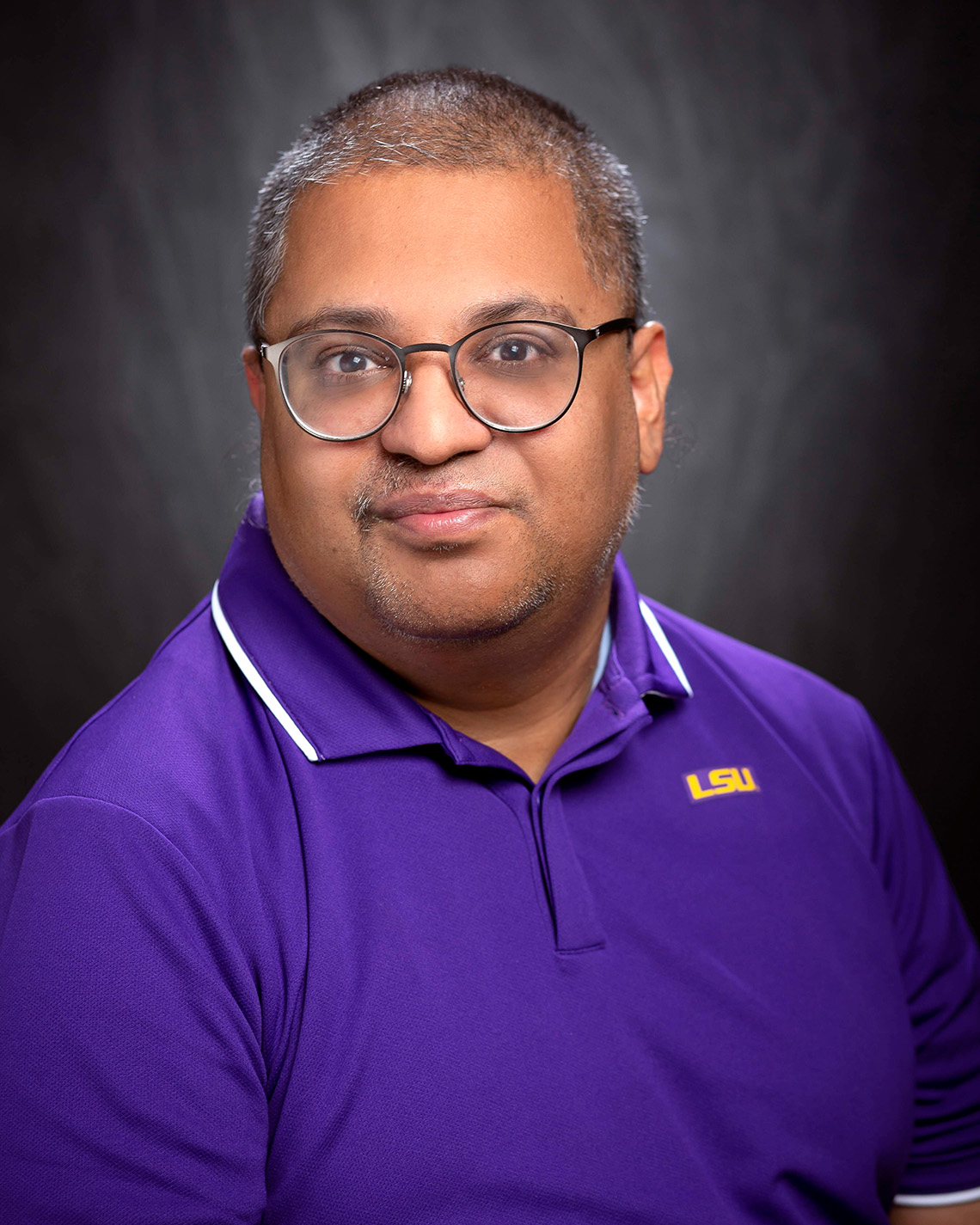
Department of Environmental Sciences Professor Supratik Mukhopadhyay
BATON ROUGE - Last August, at the height of an exceptional drought, Louisiana experienced more than 550 wildfires. The largest, the Tiger Island Fire, burned more than 31,000 acres. Wildfires like these are increasing in frequency around the globe, threatening ecosystems, infrastructure and human lives, and the ability to predict and detect them poses a critical challenge.
LSU Department of Environmental Sciences, or DES, Professor Supratik Mukhopadhyay is meeting this challenge with the power of artificial intelligence, or AI. Mukhopadhyay is working with a team of experts in AI and wildfires to build a system to predict and detect wildfires with greater accuracy.
The system is called DeepFire. It has just advanced to the next round in the Space-Based Wildfire Detection and Intelligence Track of XPrize Wildfire, a four-year, $11 million competition to develop innovative fire fighting technologies. Mukhopadhyay and his team have netted $37,500 in prize money for this round.
“Our system combines a prediction system, a detection system, and a spread modeling system that cooperate with each other,” Mukhopadhyay said. “This enables us to pinpoint our detection system to areas that are predicted to have a high risk of wildfire and deploy resources accordingly.”
According to the XPrize Wildfires website, the winners of the Space-Based Wildfire Detection & Intelligence track “will have one minute to accurately detect all fires across a landscape larger than entire states or countries, and 10 minutes to precisely characterize and report data with the least false positives to decision-makers on the ground.”
In DeepFire, systems of wildfire prediction and detection work in tandem. The system predicts locations of potential fires by examining satellite and weather station data, as well as information about previous fire behavior. It generates maps at different time scales, which fire managers may use to deploy unused resources in preparation for a blaze.
The prediction system has shown around 90 percent accuracy (4 percent more accurate compared to the existing state-of-the-art) in predicting wildfires weeks before they have started in a variety of locations that include Indonesia, California, and Alberta, Canada.
The detection system uses information on fire prone regions, weather information, including lightning strikes, and analysis of visual data to detect smoke.
It cross references this information with a predictive map in order to increase or decrease confidence that a fire is occurring.
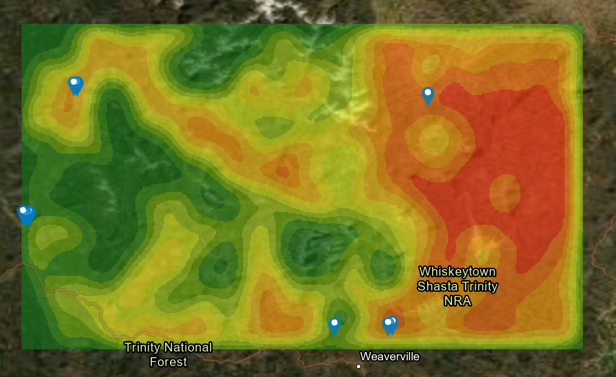
A wildfire risk map of Northern California, generated by the predictive model. Pins represent fires that occurred within two weeks after the map was generated. (All fires shown in green areas were the result of arson within city limits.)
It is able to detect eight out of ten simulated smokes, with zero false alarms making it through the successive confirmation process.
Teaching the system how to accurately identify wildfire smoke is a critical yet challenging part of the process. Smoke is highly variable in shape and size and can be easily confused with clouds. DeepFire designers have trained the machine using 50,000 space based images of smoke and fire in many different contexts.
Mukhopadhyay’s team also includes two LSU alumni, Dylan Wichman, a former STAMPS scholar who graduated in computer science, and Robert DiBiano, who studied under Mukhopadhyay while receiving his PhD in Artificial Intelligence, Machine Learning and Computer Vision.